FusionCharts will render here |
|
Hilke Bahmann (Chemistry, Wuppertal) |
Ha Quang Minh (AI, RIKEN-AIP) |
Principal Investigators
Postdoctoral researchers and Graduate students
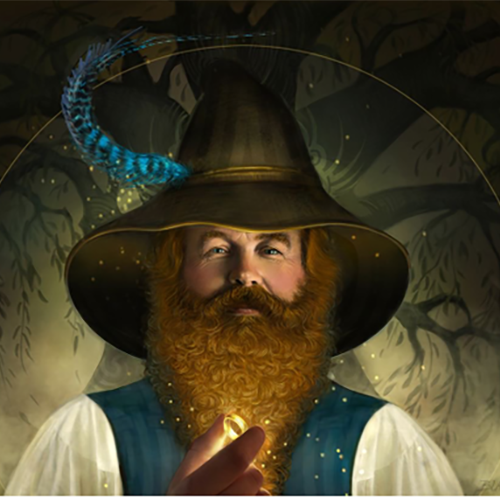
Adolfo Vargas-Jiménez
Postdoctoral Researcher
Mathematics
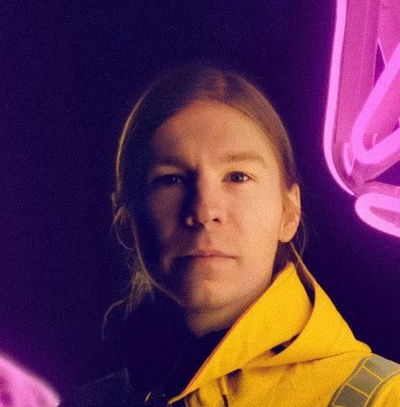
Dmitry Evdokimov
PhD student
AI for Chemistry & Physics
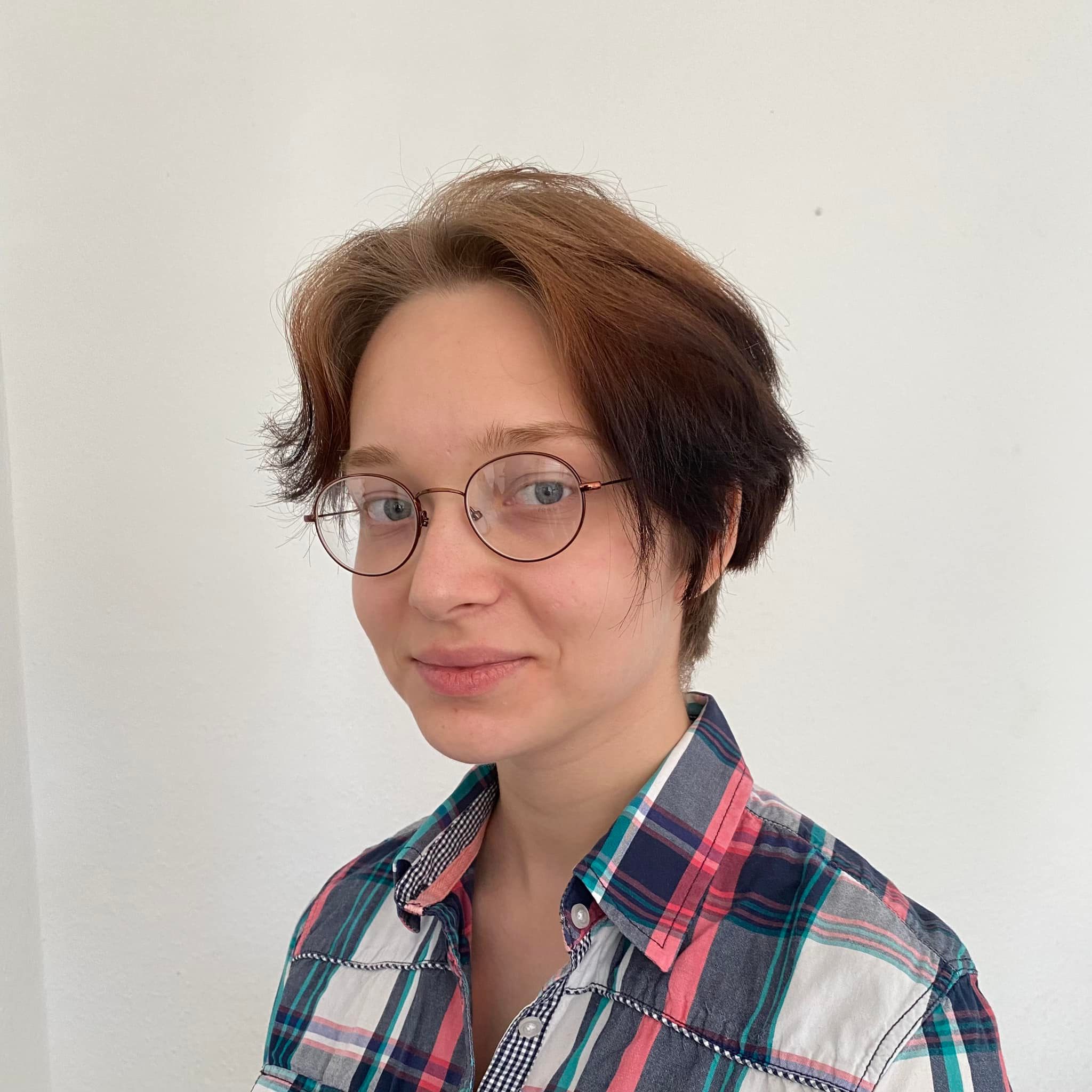
Nataliia Monina
PhD student
Math & Quantum Chemistry
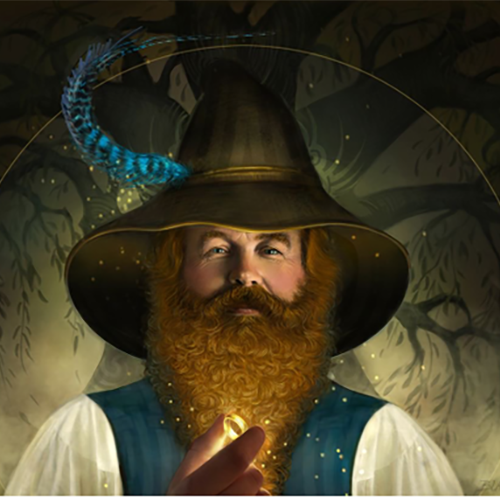
Vitalii Bielievtsov
MSc student
DTI & AI
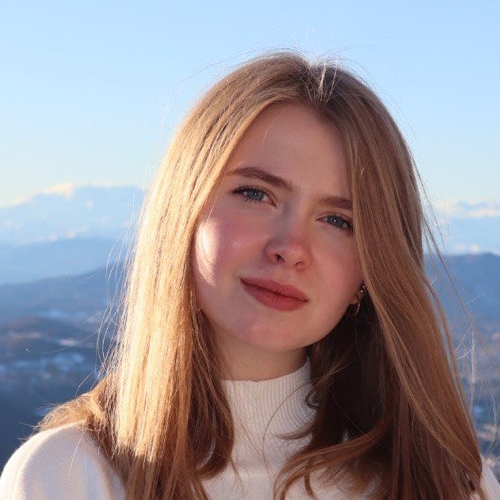
Valeria Kolesnik
MSc student (with Prof. S. Schillo)
Data Sciences
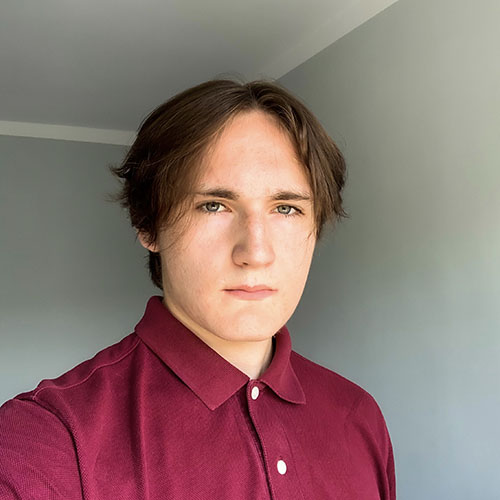
Pavlo Pelikh
MSc student
Optimal Transport & AI
Visiting Researchers and Students
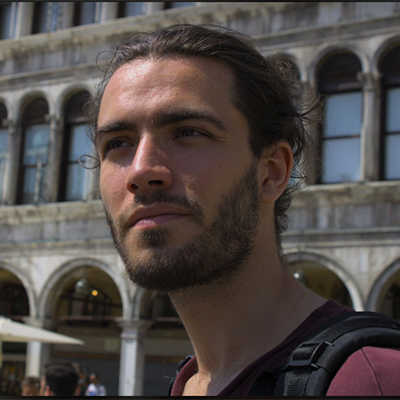
Fanch Coudreuse
PhD student (France)
Mathematics, ENS-Lyon (France)
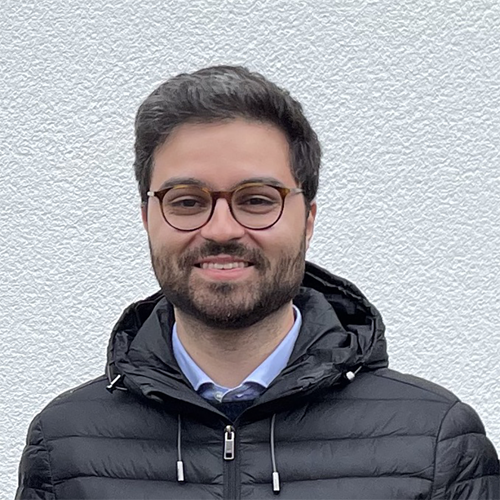
Eric Zizzi
PhD student (Italy)
Bioengineering, Politecnico di Torino
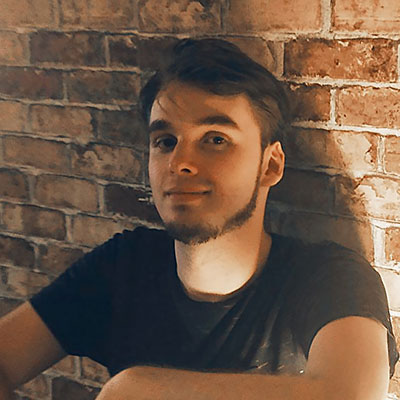
Nikita Davydov
Undergraduate (Ukraine)
Computer Sciences, Kharkiv
Undergraduate students and interns
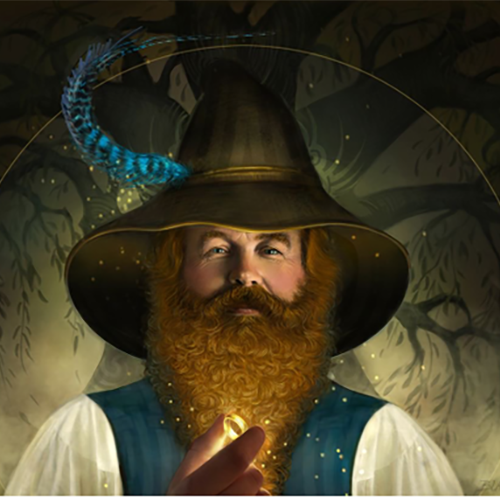
Adeline Avenido
Volunteer student
Biopharmaceutical Science
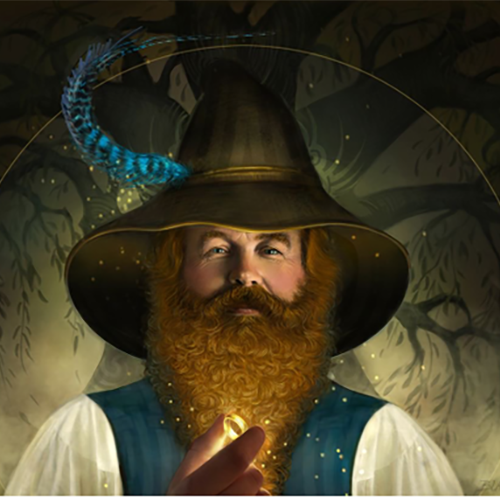
Haïda Diamouténé
Honours student
Biochemistry
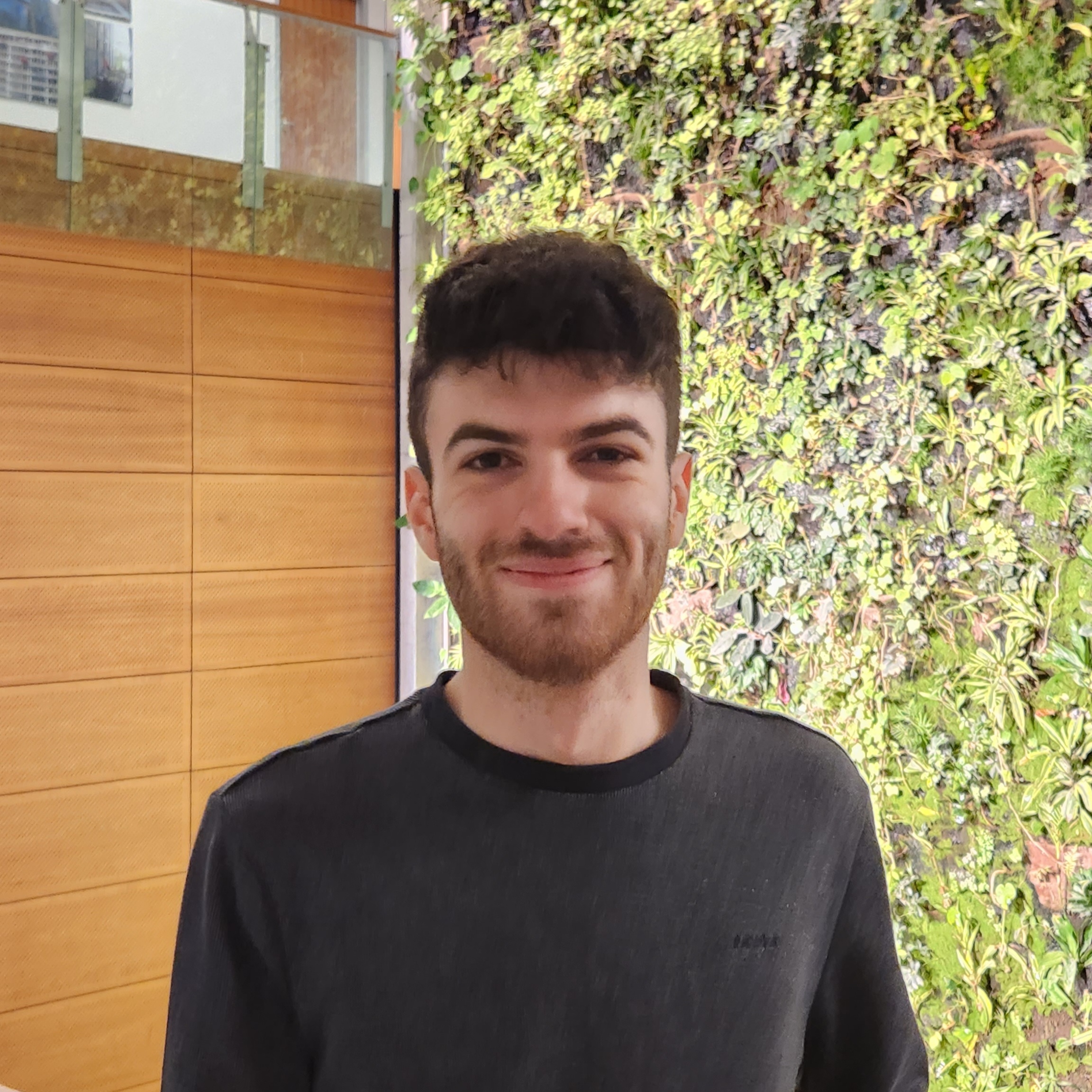
Jean Paul Kazzi
UROP student
Biotechnology

Jacqueline Kuan
Honor student
Biopharmaceutical Science
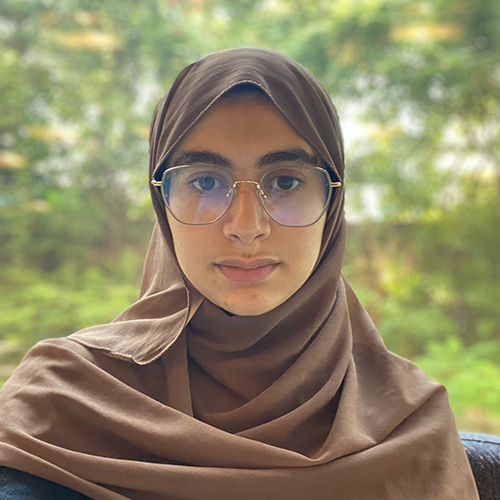
Aya Mikou
Volunteer student
Biology